W wyniku współpracy międzynarodowej KMiSD ukazał się w czasopiśmie "Energies" (IF:3.0, 140 pkt MNiSW) kolejny artykuł dotyczący badań przepływów wielofazowych: Mayet A., Fouladinia F., Hanus R., Parayangat M., Raja M.R., Muqeet M.A., Mohammed S.A.: Multiphase Flowʼs Volume Fractions Intelligent Measurement by a Compound Method Employing Cesium137, Photon Attenuation Sensor, and Capacitance-Based Sensor. Energies 17(14), 2024, 3519. (https://www.mdpi.com/1996-1073/17/14/3519)
Abstract
Multiphase fluids are common in many industries, such as oil and petrochemical, and volume fraction measurement of their phases is a vital subject. Hence, there are lots of scientists and researchers who have introduced many methods and equipment in this regard, for example, photon attenuation sensors, capacitance-based sensors, and so on. These approaches are non-invasive and for this reason, are very popular and widely used. In addition, nowadays, artificial neural networks (ANN) are very attractive in a lot of fields and this is because of their accuracy. Therefore, in this paper, to estimate volume proportion of a three-phase homogeneous fluid, a new system is proposed that contains an MLP ANN, standing for multilayer perceptron artificial neural network, a capacitance-based sensor, and a photon attenuation sensor. Through computational methods, capacities and mass attenuation coefficients are obtained, which act as inputs for the proposed network. All of these inputs were divided randomly in two main groups to train and test the presented model. To opt for a suitable network with the lowest rate of mean absolute error (MAE), a number of architectures with different factors were tested in MATLAB software R2023b. After receiving MAEs equal to 0.29, 1.60, and 1.67 for the water, gas, and oil phases, respectively, the network was chosen to be presented in the paper. Hence, based on outcomes, the proposed approach’s novelty is being able to predict all phases of a homogeneous flow with very low error.
Kolejna publikacja w czasopiśmie "Energies"
2024-07-18
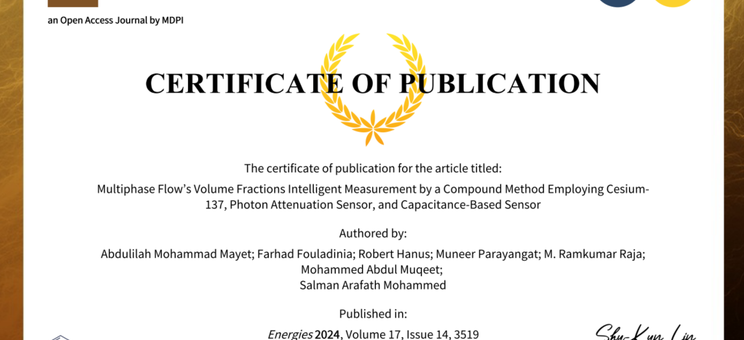